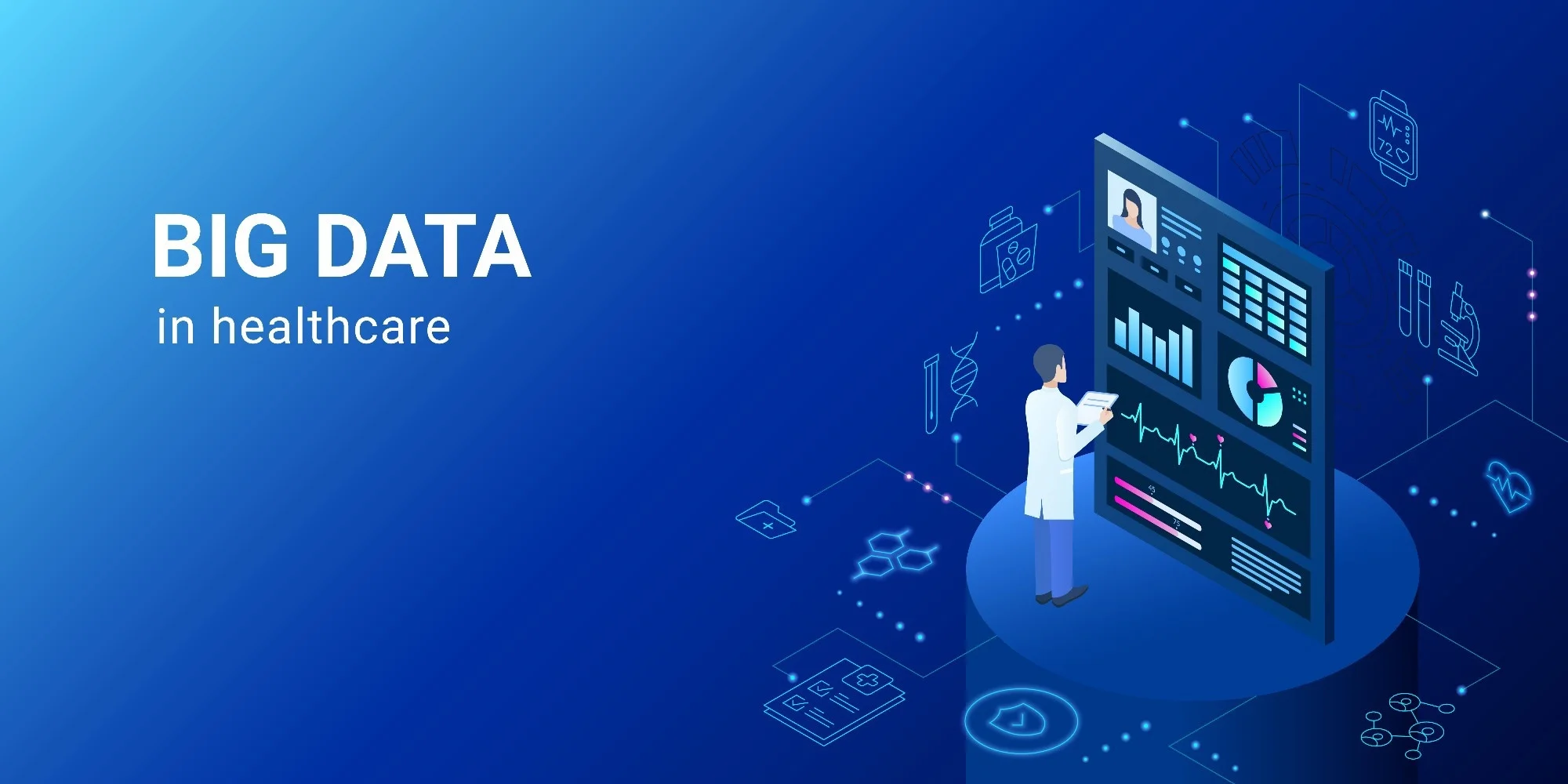
For Every rapid urbanization, global travel, and emerging infectious diseases, timely detection and response to disease outbreaks are essential for public health. With the surge in data generation, Big Data has become a transformative tool for tracking diseases and predicting outbreaks, enabling health organizations and governments to identify, contain, and respond to public health threats more effectively than ever before.
In this post, we’ll explore how Big Data analytics supports disease tracking, the technologies and methods involved, and the profound impact on healthcare and public safety.
Summary of Contents
The Importance of Disease Tracking and Outbreak Prediction
Disease tracking and outbreak prediction are crucial for preventing the spread of infectious diseases, minimizing the economic impact of pandemics, and ultimately saving lives. Traditional methods relied on healthcare records, lab reports, and in-field surveys, often resulting in delays and limited scope. Now, Big Data analytics allows health organizations to collect, analyze, and interpret vast amounts of data from diverse sources in real-time.
This shift has revolutionized the response to disease outbreaks by:
- Improving early detection through real-time data analysis,
- Enhancing predictive accuracy by integrating multiple data sources, and
- Enabling a faster, more targeted response to contain outbreaks.
Key Data Sources for Disease Tracking and Prediction
- Electronic Health Records (EHRs)
EHRs offer a rich source of data, including patient demographics, diagnosis, and treatment history. Analyzing EHRs can help identify early signs of outbreaks based on symptom patterns, disease prevalence, and unusual trends. (Ref: Telemedicine & Remote Monitoring) - Social Media and Search Engine Data
Platforms like Twitter, Facebook, and Google search logs provide insights into public sentiment, symptom searches, and regional spikes in health-related queries. For example, a rise in searches for “flu symptoms” in a particular area could signal the onset of an outbreak. - Public Health Surveillance Systems
Organizations such as the World Health Organization (WHO) and Centers for Disease Control and Prevention (CDC) operate surveillance systems that collect and share data on reportable diseases, case counts, and emerging health threats. - Mobile and Wearable Data
Data from mobile devices and wearables can provide real-time insights into people’s health status, locations, and mobility patterns, offering clues to how diseases may spread within communities or across regions. - Environmental Data
Environmental factors like temperature, humidity, and pollution levels can impact disease transmission rates. For instance, certain mosquito-borne diseases thrive in warmer climates, making weather data valuable for predicting outbreaks. - Genomic and Laboratory Data
Genome sequencing helps identify and track virus mutations, aiding in the study of how infectious diseases evolve and spread. Outbreak Prediction Genomic data also helps scientists understand the relationship between various strains and predict potential impacts.
How Big Data Analytics Facilitates Disease Tracking
- Real-Time Data Analysis
Real-time data analysis helps health officials respond more quickly to potential outbreaks. Data processing tools aggregate and analyze incoming data continuously, allowing public health agencies to detect unusual patterns or anomalies that could indicate disease spread. - Predictive Modeling
Predictive models leverage historical data, machine learning algorithms, and epidemiological models to forecast future disease outbreaks. These models consider various factors, such as population density, travel patterns, and seasonal trends, to predict when and where an outbreak might occur. - Geospatial Analysis
Geospatial analytics tools overlay disease data with geographical information, enabling health organizations to monitor the spatial distribution of cases and identify outbreak hotspots. Maps and visualizations can display disease progression in real-time, offering insights for targeted interventions. - Sentiment and Trend Analysis
Using Natural Language Processing (NLP) to analyze social media and search trends, health officials can gauge public concern and symptom reporting. NLP can detect specific health-related keywords or unusual clusters of complaints, providing a valuable, crowdsourced view of potential outbreaks. - Mobility Pattern Analysis
Mobility data from phones and transport networks allows analysts to track how people move between regions, identifying routes that diseases might follow. Outbreak Prediction By understanding human mobility patterns, health organizations can implement containment measures, like travel restrictions or targeted awareness campaigns. - Disease Spread Simulations
Simulation models, powered by AI, create hypothetical disease scenarios to study how an outbreak might unfold under different conditions. These simulations allow public health experts to prepare response plans, test intervention strategies, and evaluate containment measures.
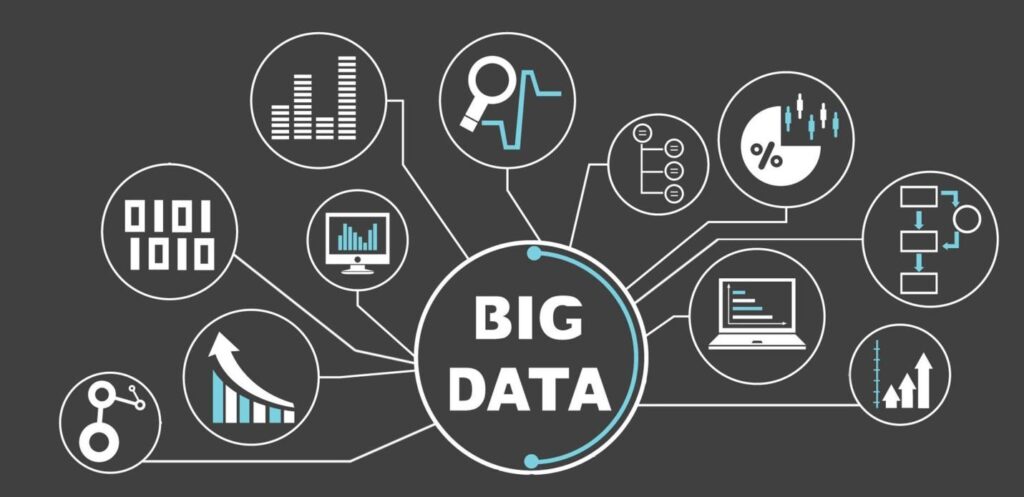
Technologies Enabling Big Data Disease Tracking
- Machine Learning and AI
Machine learning algorithms analyze complex datasets, identify patterns, and predict outcomes with greater accuracy. AI-powered systems can process vast amounts of information from various sources, helping detect diseases and predict outbreaks more effectively. - Cloud Computing
Cloud-based platforms offer scalable storage and processing power, essential for handling Big Data. Cloud solutions also enable data-sharing between organizations, facilitating collaboration in tracking diseases across borders. - Geographical Information Systems (GIS)
GIS software visualizes disease data on maps, making it easier to monitor outbreaks geographically. GIS applications provide insights into where resources are needed most, helping prioritize containment efforts. - Natural Language Processing (NLP)
NLP processes and interprets unstructured data from social media, news articles, and online forums, revealing public sentiment and symptoms reported in real-time. (Ref: Drive Business Success with Custom NLP Solutions & Implementation) - Internet of Things (IoT)
IoT devices, such as health monitors and environmental sensors, contribute real-time data on health and environmental conditions, providing crucial information for disease tracking and analysis.
Real-World Applications of Big Data in Disease Tracking
- COVID-19 Monitoring and Response
During the COVID-19 pandemic, Outbreak Prediction Big Data played a significant role in monitoring infection rates, contact tracing, and predicting hotspots. Health organizations leveraged data from mobile apps, social media, and EHRs to track and contain the spread of the virus. AI models predicted disease trajectories, helping governments make data-driven decisions on lockdowns and healthcare resource allocation. - Predicting Seasonal Flu Trends
Flu outbreaks are monitored using predictive analytics and social media trends. Outbreak Prediction Google Flu Trends, for instance, used search data to estimate flu activity in specific regions, offering insights weeks ahead of traditional health reports. Although discontinued, similar models continue to provide early warnings for flu outbreaks. - Tracking Malaria in Endemic Regions
In Africa, Big Data analytics helps track malaria outbreaks by combining climate data, Outbreak Prediction population movement, and historical disease data. This information assists in targeted interventions, such as distributing mosquito nets and scheduling preventive measures based on high-risk areas. - Early Detection of Dengue Fever
Public health agencies in tropical regions use data on weather, mosquito populations, and historical case data to predict and mitigate dengue outbreaks. Outbreak Prediction Real-time environmental data helps anticipate outbreaks, enabling preventive measures like insecticide spraying in high-risk areas.
Benefits of Big Data in Disease Tracking and Outbreak Prediction
- Rapid Response
Real-time data analysis enables health authorities to respond swiftly to emerging outbreaks, containing the spread before it escalates. - Improved Accuracy
By integrating diverse data sources, predictive models deliver more accurate forecasts, allowing public health officials to act proactively rather than reactively. - Resource Optimization
Disease tracking helps allocate healthcare resources more effectively, ensuring that critical supplies like vaccines, hospital beds, and personnel are available where they’re needed most. - Enhanced Collaboration
Big Data platforms facilitate information-sharing between countries and organizations, fostering global collaboration in tracking and responding to health threats. - Public Awareness and Engagement
With access to real-time data and insights, health agencies can launch targeted awareness campaigns, educating the public on preventive measures and reducing the spread of disease.
Final Thoughts : A New Era of Disease Control with Big Data
Big Data analytics has ushered in a new era of disease tracking and outbreak prediction, offering insights that can save lives and protect communities. Outbreak Prediction By harnessing data from EHRs, social media, mobility patterns, and environmental sources, health organizations can detect disease trends early, respond proactively, and ultimately improve public health outcomes.
As technology continues to evolve, the potential for Big Data in disease tracking will expand, helping us tackle emerging health challenges with unprecedented speed and precision. For governments, Outbreak Prediction healthcare providers, and researchers, investing in Big Data is essential for building a healthier, safer future for everyone.