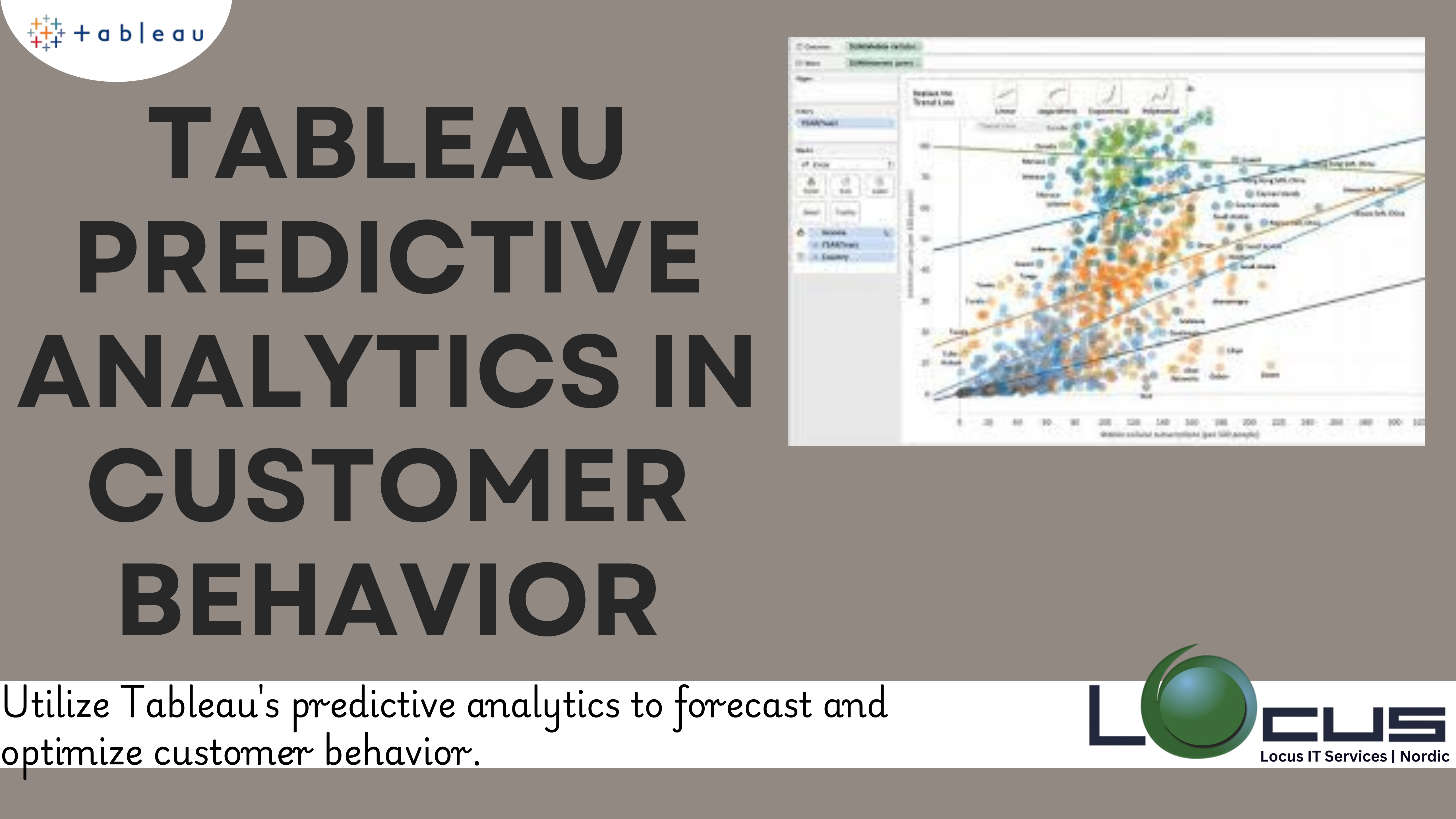
Understanding customer behavior is pivotal for businesses aiming to thrive in highly competitive markets. Predictive analytics has emerged as a game-changing strategy, enabling companies to anticipate customer needs, improve experiences, and foster loyalty. Tableau, a leading business intelligence and data visualization tool, plays a significant role in transforming raw data into actionable insights that drive Tableau predictive analytics in customer behavior.
This blog explores Tableau’s contribution to predictive customer behavior analytics, its integration with machine learning models, and its impact on business strategy and customer relationship management.
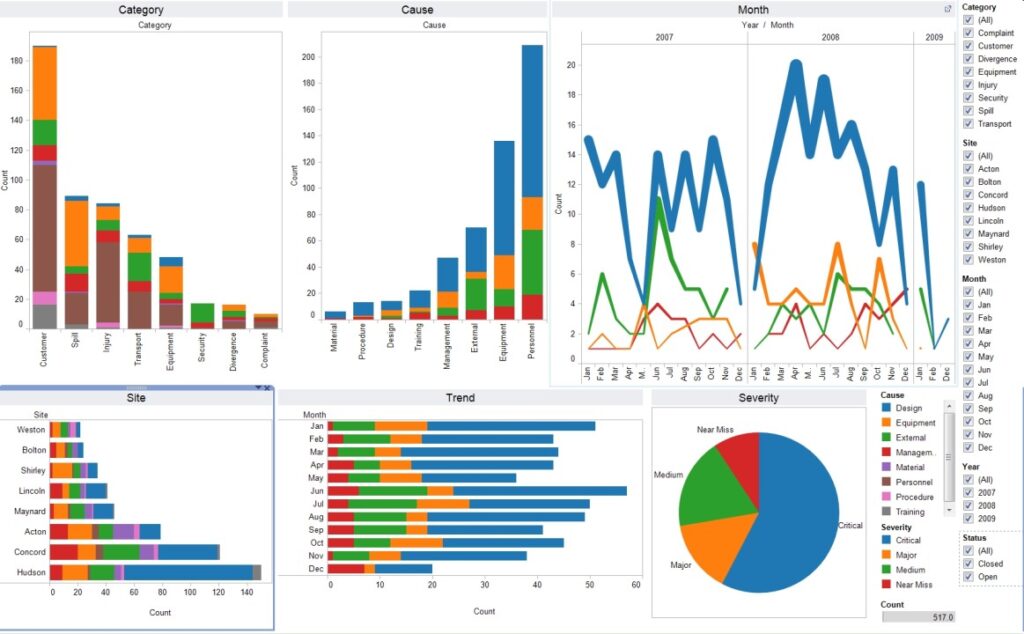
Importance of Tableau Predictive Analytics in Customer Behavior
Understanding what drives customer behavior is not new; businesses have always sought insights to improve marketing strategies, product development, and service delivery. However, Tableau predictive analytics has redefined this understanding by leveraging historical and real-time data to forecast future trends and behaviors.
Predictive customer behavior analytics can:
Anticipate customer needs: Businesses can recommend products or services proactively.
Enhance personalization: Tailored marketing campaigns resonate better with customers.
Improve customer retention: Predict churn rates and address concerns before customers leave.
Optimize pricing strategies: Predict demand patterns for better inventory and pricing decisions.
(Ref: Tableau ESG Reporting: Transforming Data with Analytics)
Tableau: A Powerhouse for Data Visualization
Tableau excels as a visualization platform, making complex datasets easy to interpret through dynamic dashboards and interactive graphics. With its user-friendly interface, Tableau enables even non-technical stakeholders to gain valuable insights into data trends.
Key Features That Support Tableau Predictive Analytics
Dynamic Dashboards: Real-time data updates allow for quick decision-making.
Integration Capabilities: Tableau integrates seamlessly with Tableau predictive analytics tools, databases, and machine learning models like Python, R, and TensorFlow.
Advanced Calculations: Create custom measures to analyze trends and patterns effectively.
User-Friendly Interface: Drag-and-drop features make it accessible to users of varying expertise levels.
Guiding Tools: Build narratives that communicate the data-driven predictions clearly to stakeholders.
How Tableau Enhances Predictive Customer Behavior Analysis
Tableau predictive analytics on its own but serves as a critical enabler by presenting data in an actionable format. When paired with machine learning and predictive models, Tableau helps businesses harness the power of their data.
1.Data Preparation and Exploration
Tableau simplifies the process of data cleaning and preparation. Users can import datasets from various sources, such as CRMs, ERPs, social media platforms, and website analytics tools.
Using Tableau Prep, businesses can organize, clean, and combine datasets to form a robust foundation for analysis. This preprocessing ensures high-quality inputs for predictive models, which in turn leads to more reliable predictions.
2.Integration with Machine Learning Tools
Tableau’s ability to connect with advanced tools like Python and R enhances its analytical power. By integrating Tableau with predictive models, businesses can:
-Forecast customer churn using historical interaction data.
-Identify cross-sell and upsell opportunities by analyzing buying patterns.
-Predict product demand based on seasonal and market trends.
For example, a retail company can use Python to build a predictive model for customer purchase behavior and visualize the results in Tableau for actionable insights.
3.Real-Time Insights
In today’s fast-paced world, real-time data is essential. Tableau provides live data visualization by connecting directly to databases. For instance, businesses can monitor how customers are engaging with a website or app in real-time and adapt their strategies on the fly.
4.Segmentation and Personalization
Segmentation is a crucial aspect of predictive customer behavior. Tableau enables businesses to create granular customer segments based on predictive insights, such as:
-Demographic data
-Purchase history
-Interaction frequency
-Predicted lifetime value
These segments can be used to design personalized marketing campaigns or loyalty programs, increasing customer satisfaction and retention.
Case Studies
Case Study 1: E-commerce Personalization
An online retail platform used Tableau to visualize customer browsing and purchase data. By integrating with a Tableau predictive analytics tool, the company identified which customers were likely to abandon their carts. Using Tableau dashboards, the team could monitor real-time behavior and launch targeted email campaigns, recovering 25% of abandoned carts.
Case Study 2: Predicting Churn in Telecommunications
A telecommunications company analyzed call center data and customer complaints in Tableau. After integrating predictive models, they identified customers with a high likelihood of switching providers. By offering customized retention plans, they reduced churn rates by 15%.
Case Study 3: Financial Services
A bank used Tableau to analyze customer transaction data. By predicting which customers were likely to opt for premium services, they launched targeted campaigns, resulting in a 20% increase in premium account sign-ups.
Benefits of Using Tableau for Predictive Customer Behavior
Improved Decision-Making
By presenting data in an easy-to-understand format, Tableau ensures that decision-makers can act on insights quickly and confidently.
Enhanced Collaboration
Tableau’s sharing capabilities allow teams across departments to collaborate effectively on customer behavior strategies for Tableau predictive analytics.
Scalability
Whether for small businesses or large enterprises, Tableau adapts to different scales and complexities of data.
Cost Efficiency
Integrating predictive models with Tableau often eliminates the need for expensive, standalone analytics tools.
Greater Customer Understanding
Detailed visualizations help businesses understand customer behavior more deeply, leading to better engagement strategies.
Challenges and How Tableau Addresses Them
While Tableau is a powerful tool, there are challenges in using it for Tableau predictive analytics:
Data Complexity: Integrating disparate datasets can be time-consuming. Tableau addresses this through Tableau Prep and robust integration capabilities.
Skill Requirements: Tableau requires users to have some understanding of data analysis. With extensive training resources and a supportive community, this challenge is manageable.
Predictive Modeling: Tableau isn’t a predictive tool itself but complements models built in tools like Python or R. Its integration features bridge this gap effectively.
Future of Tableau Predictive Analytics
The future of Tableau in predictive customer behavior looks promising. With advancements in AI and machine learning, Tableau is expected to integrate even more seamlessly with predictive tools, offering built-in predictive features like AI-driven forecasts and advanced anomaly detection.
Moreover, as businesses increasingly prioritize customer-centric strategies, Tableau will continue to play a vital role in making Tableau predictive analytics accessible, actionable, and impactful.
Final Thoughts
Predictive customer behavior analytics empowers businesses to stay ahead of the curve by anticipating customer needs and delivering personalized experiences. Tableau’s ability to transform complex data into meaningful visualizations makes it an indispensable tool in this domain. By integrating with predictive models and providing real-time, actionable insights, Tableau ensures businesses can make informed decisions, improve customer satisfaction, and drive growth.
As the digital landscape evolves, the role of tools like Tableau in predictive analytics will only grow, shaping the future of customer engagement and business success. For organizations looking to leverage their data effectively, Tableau offers the perfect blend of functionality, flexibility, and foresight.