Machine Learning Support Services (MLSS) are revolutionizing conventional support systems into dynamic, quick, highly responsive systems in the fast changing sector of IT support. These services are redefining what it means to support by leveraging artificial intelligence (AI) and machine learning (ML), therefore enabling companies to stay up with changing customer expectations and technical developments.
Machine Learning Support Services
Numerous cutting-edge apps that are intended to increase operational efficiency and support service accuracy include machine learning into IT support systems. ML is being used to improve service delivery in a number of ways, including real-time anomaly detection, predictive maintenance, and automated ticketing systems.
Automated Ticketing and Response Systems: ML algorithms can automatically respond to initial customer questions, classify issues according to their urgency, and forward tickets to the relevant human agents by utilizing natural language processing (NLP). This improves overall efficiency by expediting the resolution process and freeing up support professionals to work on more difficult issues.
Predictive Maintenance: Machine learning algorithms examine data patterns to anticipate possible system breakdowns before they materialize. Proactive maintenance is made possible by this predictive capabilities, which drastically lowers operating expenses and downtime. Organizations may provide a more seamless, continuous service by resolving problems before they interfere with company activities.
Real-Time Anomaly Detection: By using machine learning methods to continuously monitor systems, anomalous behaviors that can point to possible threats or failures can be quickly identified. Especially in industries where security and dependability are critical, this immediate identification is essential for averting significant interruptions and preserving system integrity.
There are various essential processes involved in deploying machine learning support services, ranging from data gathering and model training to integration and continuous management:
Data collection: The first stage is compiling a large amount of data from multiple sources, such as system logs, user interactions, and performance measurements. Training strong machine learning models that can precisely anticipate and react to support scenarios requires this data.
Model Training: To identify patterns and anomalies, supervised learning is used to train machine learning models using the gathered data. As fresh data becomes available, these models continue to learn and improve their reactions and predictions.
Integration: It takes careful preparation and execution to incorporate ML models into current IT support frameworks. This procedure must guarantee that machine learning support services integrate easily with other IT infrastructure elements, improving tools without interfering with current workflows.
Continuous Optimization: To guarantee that machine learning support services systems operate at their best, they need to be continuously observed. To respond to emerging issues and shifts in user behavior or system architecture, frequent modifications and upgrades are required.
There are several advantages of using Machine Learning Support Services:
Increased Productivity: Routine task automation greatly reduces reaction times and frees up human agents to handle more complicated problems, increasing total productivity.
Decreased Downtime: By guaranteeing that any problems are fixed before they have an impact on operations, predictive maintenance capabilities reduce system downtime.
Increased Customer Satisfaction: Proactive problem-solving and quicker replies lead to happier and more devoted customers.
Cost Savings: Businesses can save a lot of money by automating support jobs and cutting down on downtime.
Notwithstanding the advantages, there are certain difficulties in incorporating machine learning support services:
Data privacy and security: To prevent breaches and guarantee adherence to data protection laws, handling substantial amounts of sensitive data necessitates strong security measures.
Skill Gap: Businesses must spend money on training and development to close the skills gap in order to meet the high demand for professionals with expertise in machine learning support services
Complexity of Integration: It can be difficult and resource-intensive to integrate machine learning technology into current IT systems.
It is anticipated that machine learning technologies will become more deeply integrated into IT support services as they develop, bringing with them increasingly complex capabilities and innovations. More widespread automation of complex jobs, deeper personalization of support services, and improved predictive analytics are possible future advancements. The field of IT assistance will probably undergo further change as a result of these developments, becoming more proactive, individualized, and effective.
In the current digital era, machine learning support services are not just an operational improvement but also a strategic requirement. ML is establishing a new benchmark for IT assistance by automating replies, anticipating system breakdowns, and identifying anomalies instantly. Companies that use these technologies can gain a major competitive edge by increasing customer happiness, cutting expenses, and improving operational efficiency. Businesses’ approaches to IT support will continue to be transformed by ML technologies as they advance, becoming more intelligent, efficient, and quick.
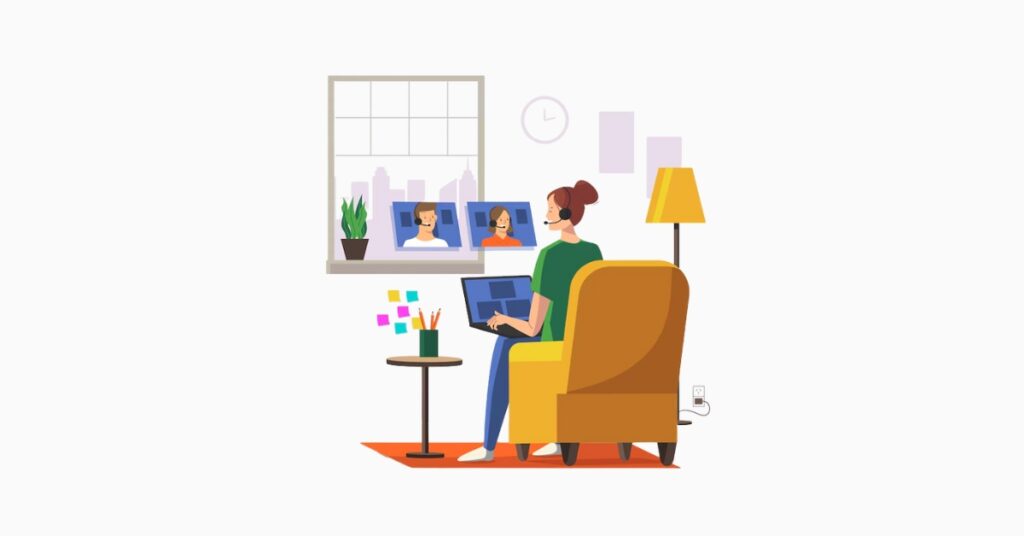