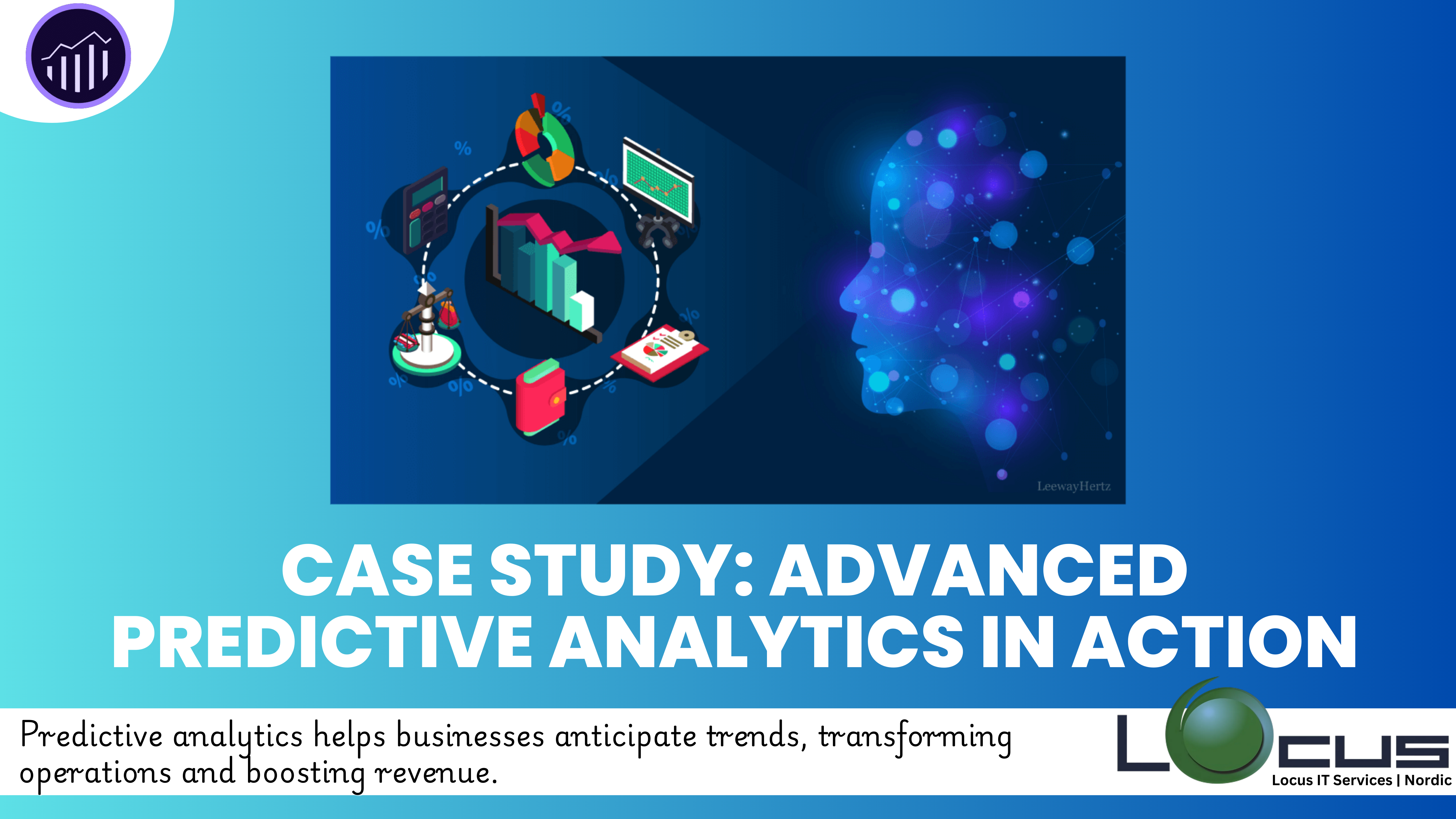
Every businesses must move beyond reactive strategies and embrace predictive analytics to anticipate trends, behaviors, and opportunities. This case study explores how a retail company successfully implemented advanced predictive analytics to transform its operations, enhance customer satisfaction, and boost revenue. (Ref: Automating Predictive Models with Adobe Sensei)
The Challenge: Addressing Customer Retention and Inventory Issues
Our subject, a mid-sized online retailer, faced two critical challenges:
- Customer Retention: The company was struggling with declining customer retention rates, with many first-time buyers failing to return.
- Inventory Management: Stockouts and overstock situations were affecting profitability and customer satisfaction.
The retailer sought a data-driven approach to better understand customer behavior and optimize inventory management.
The Solution: Implementing Advanced Predictive Analytics
To address these challenges, the company partnered with a predictive analytics consultancy. Here’s how they implemented a solution:
1. Data Consolidation and Preparation
The first step was to consolidate data from various sources, including:
- Customer purchase histories
- Website behavior data
- Social media interactions
- External factors like seasonal trends and market data
The team used a predictive analytics platform capable of handling large datasets and performing real-time analysis.
2. Machine Learning Models
Machine learning algorithms were developed to address specific objectives:
- Customer Churn Prediction: A model identified patterns in behavior that indicated a likelihood of churn, such as reduced website visits or abandoned carts.
- Demand Forecasting: Another model analyzed historical sales data and seasonal trends to predict future inventory needs.
3. Real-Time Insights and Automation
The advanced predictive analytics platform provided real-time dashboards and automated alerts, allowing the team to act on insights quickly. For example, when a customer was flagged as likely to churn, the system automatically triggered personalized retention campaigns.
Key Results Achieved
Within six months of implementing advanced predictive analytics, the retailer experienced remarkable results:
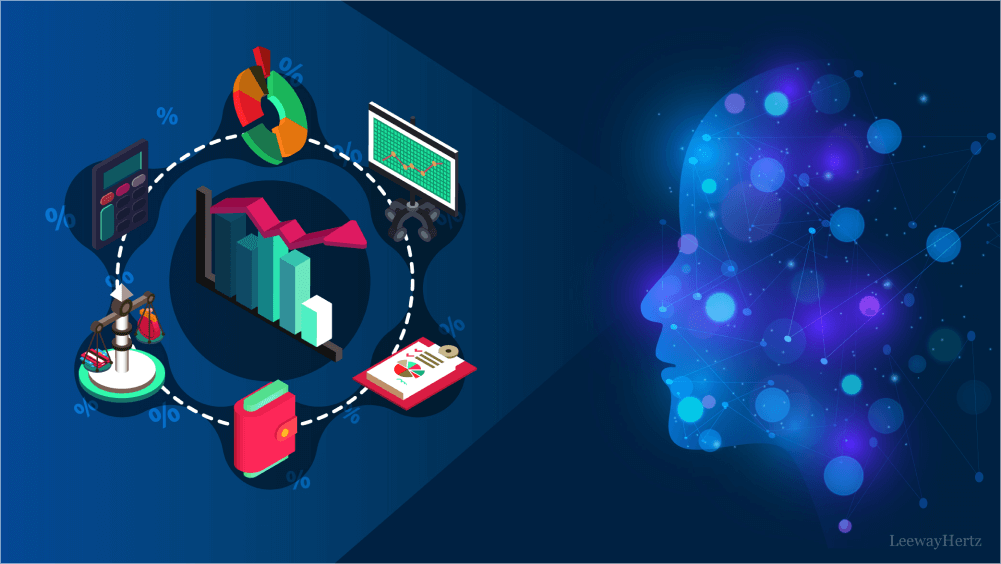
1. Improved Customer Retention
By identifying at-risk customers and launching personalized email and discount campaigns, the company increased retention rates by 20%. Personalized experiences, advanced predictive analytics such as product recommendations based on browsing history, further improved customer satisfaction.
2. Optimized Inventory Management
Accurate demand forecasts reduced stockouts by 30% and overstock situations by 25%. The company saved significantly on storage costs and avoided lost sales due to unavailable products.
3. Enhanced Marketing ROI
Advanced predictive analytics enabled the marketing team to focus on high-value customers and effective campaigns. As a result, marketing ROI increased by 40%, as fewer resources were wasted on ineffective strategies.
4. Increased Revenue
With improved retention and optimized inventory, overall revenue grew by 15% within the first year of implementation.
Lessons Learned from the Case Study: A Detailed Explanation
- Data Quality is Crucial
Predictive analytics relies heavily on the quality of the data being analyzed. Poor data—such as incomplete, outdated, or inconsistent information—can lead to inaccurate predictions and misguided decisions. Investing in proper data collection, cleaning, and integration processes ensures that the data used for analytics is accurate, relevant, and comprehensive.- Example: In the case study, the retailer consolidated customer purchase histories, website behavior data, and external factors to create a unified and reliable dataset. This clean data formed the foundation for accurate predictive models.
- Key Takeaway: Without high-quality data, even the most sophisticated predictive tools cannot deliver meaningful insights.
- Collaboration Across Teams
Predictive analytics is not an isolated task for data scientists or IT teams—it is a collaborative effort that involves multiple departments. For example:- Marketing Teams use predictive insights to create personalized campaigns.
- Operations Teams rely on demand forecasts to manage inventory effectively.
- IT Teams ensure the technical infrastructure supports data collection, integration, and analysis.
In the case study, these teams worked together to implement actionable insights derived from predictive models. - Key Takeaway: Aligning cross-departmental efforts ensures that insights from predictive analytics are not just theoretical but are effectively acted upon.
- Start Small and Scale
When implementing predictive analytics, it’s often beneficial to focus on a few specific use cases rather than trying to address all challenges at once.- In the case study, the retailer began with two well-defined problems: customer retention and inventory management. By achieving measurable success in these areas, they built confidence and expertise to scale predictive analytics to broader applications, such as pricing optimization and product development.
- Key Takeaway: Starting small allows businesses to test the feasibility of predictive analytics, refine their processes, and scale strategically over time.
- Real-Time Analytics Makes a Difference
Predictive analytics is most impactful when insights are delivered in real-time, enabling businesses to respond quickly and effectively to emerging opportunities or challenges.- In the case study, real-time dashboards and alerts allowed the retailer to take immediate actions, advanced predictive analytics such as launching personalized retention campaigns or addressing inventory shortages before they affected customer satisfaction.
- Key Takeaway: Businesses that act on real-time insights gain a competitive edge, as they can adapt to changing market conditions and customer behaviors more swiftly than their competitors.
Final Thoughts
This case study highlights the transformative potential of advanced predictive analytics. By leveraging data and machine learning, the retailer was able to address critical challenges, enhance customer experiences, and achieve sustainable growth.
Whether you’re a small business or a large enterprise, advanced predictive analytics offers actionable insights that can drive smarter decisions and better outcomes. Embrace this technology today to future-proof your business and stay ahead in a rapidly evolving market.