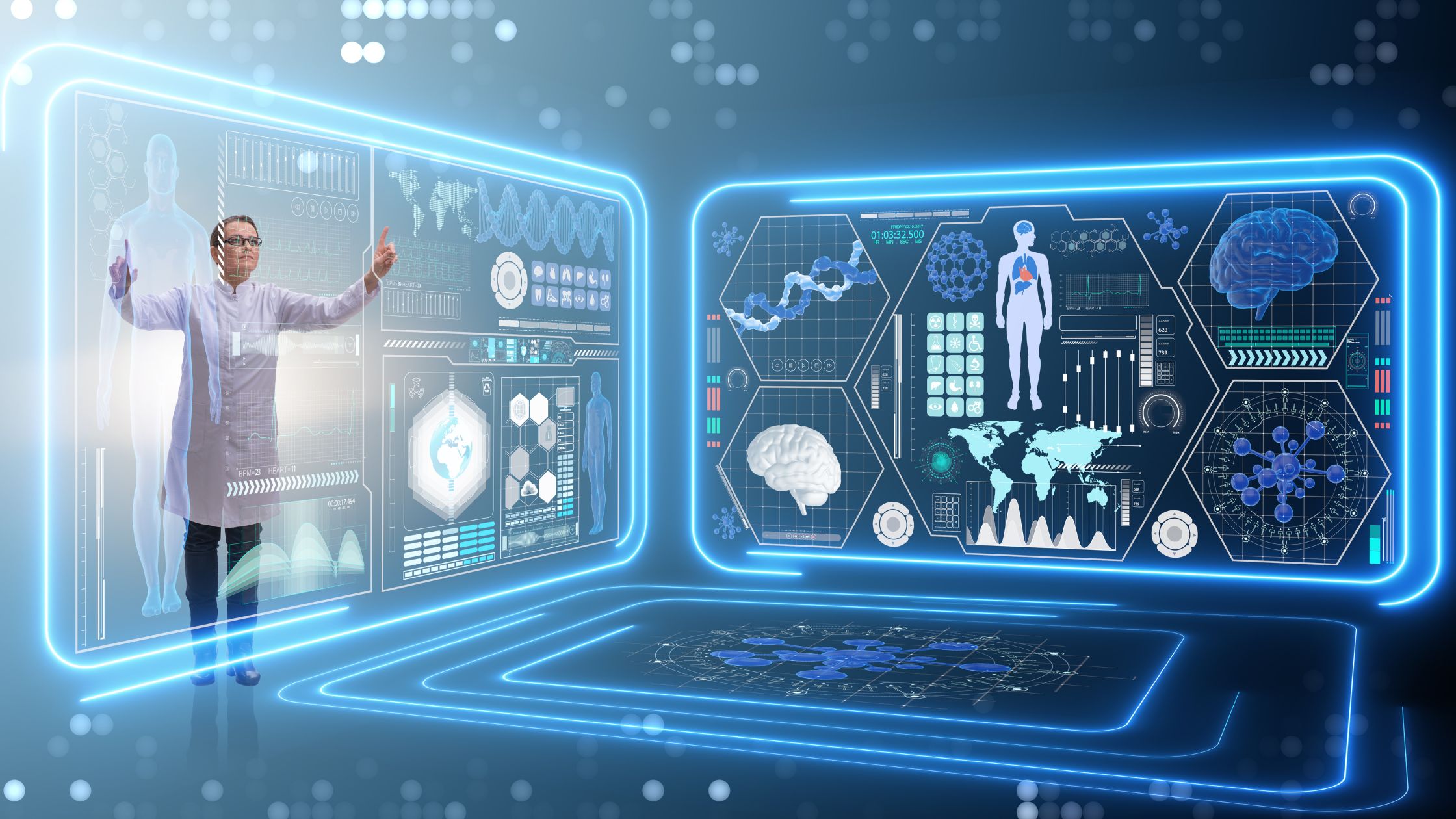
Healthcare is at the forefront of a technological revolution, driven by advancements in Big Data and Diagnosis with Machine Learning (ML). These innovations are transforming how medical professionals diagnose diseases, predict outcomes, and tailor treatments to individuals. Traditional diagnostic methods, while effective, often rely on limited data and may be prone to human error. By harnessing the power of machine learning, doctors can now analyze vast amounts of patient data to make faster, more accurate diagnoses, leading to better patient outcomes.
In this blog post, we’ll explore how machine learning is improving diagnosis in healthcare, the benefits it brings to medical practice, and how it’s reshaping the future of healthcare.
Summary of Contents
What is Machine Learning in Healthcare?
Machine learning, a subset of artificial intelligence (AI), involves training algorithms to recognize patterns and make decisions based on data. In healthcare, machine learning models analyze massive datasets, including electronic health records (EHRs), medical images, genomic data, and even patient histories, to identify patterns and anomalies that might not be immediately apparent to the human eye.
Machine learning can be used for:
- Predicting disease risk
- Diagnosing conditions
- Monitoring patient progress
- Recommending treatment plans
By processing vast amounts of data, machine learning models can detect subtle patterns and correlations, enabling more accurate and timely diagnoses.
Key Areas Where Machine Learning is Enhancing Diagnosis
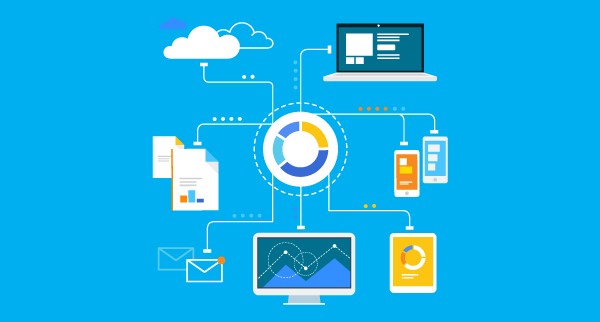
- Medical Imaging Diagnosis
One of the most significant applications of machine learning in healthcare is the analysis of medical images, such as X-rays, MRIs, CT scans, and ultrasounds. Traditionally, interpreting medical images required expert radiologists, but the sheer volume of images can lead to missed diagnoses and inconsistent readings. Diagnosis with Machine Learning, especially deep learning algorithms, have proven highly effective in analyzing medical images. These algorithms are trained to recognize specific patterns or abnormalities within images, such as tumors, fractures, or infections.- Impact: ML-driven diagnostic tools can provide radiologists with more accurate readings, reducing the risk of human error, speeding up diagnosis, and improving patient outcomes. For example, Google Health has developed an AI model that can detect breast cancer more accurately than human radiologists.
- Predictive Analytics for Disease Risk
Predictive analytics uses machine learning to forecast the likelihood of a patient developing a particular condition, based on their Diagnosis with Machine Learning history, lifestyle factors, genetic data, and other relevant information. For example, heart disease, diabetes, and cancer can often be predicted using predictive models trained on large datasets of patient records. (Ref: Big Data Architecture: Building the Foundation for Data-Driven Success)- Impact: By predicting the risk of disease before symptoms appear, doctors can intervene earlier, enabling preventive measures that can significantly reduce morbidity and mortality rates. For instance, ML models can predict a patient’s risk of heart attack by analyzing factors like blood pressure, cholesterol levels, and genetic predispositions.
- Genomics and Personalized Medicine
In genomics, machine learning helps analyze large-scale genetic data to identify mutations, genetic markers, or rare diseases that may be difficult to detect using traditional methods. Diagnosis with Machine Learning can identify patterns in a person’s DNA that suggest a higher risk of developing certain conditions or diseases, such as cancer, Alzheimer’s, or genetic disorders.- Impact: By using machine learning to analyze genetic data, healthcare providers can offer personalized treatment plans that target specific genetic profiles, leading to more effective and individualized care. This is particularly important in fields like precision medicine, where treatments are tailored to the unique genetic makeup of each patient.
- Natural Language Processing (NLP) for Clinical Notes
A large portion of patient data resides in unstructured formats, such as clinical notes written by doctors during patient visits. These notes contain valuable information about patient symptoms, history, and treatment progress but are often difficult to analyze manually. Natural Language Processing (NLP), a branch of machine learning, enables computers to understand, interpret, and process human language. (Ref: Natural Language Processing)- Impact: By applying NLP algorithms to clinical notes, healthcare providers can extract important insights, detect early signs of diseases, and even identify patterns or trends that would be difficult to spot otherwise. For example, NLP can help detect symptoms of depression or mental health conditions based on a patient’s interactions with doctors.
- Predicting Patient Outcomes
Machine learning is also playing a pivotal role in predicting patient outcomes. By analyzing historical data from patients with similar conditions, Diagnosis with Machine Learning can forecast how a particular patient is likely to respond to a treatment, what the possible complications are, and the likelihood of recovery.- Impact: These predictions help doctors develop more accurate treatment plans, anticipate challenges, and monitor the progress of patients. For instance, ML models are used to predict the survival rate of cancer patients, or the likelihood of readmission to the hospital after surgery, enabling better decision-making.
Benefits of Diagnosis with Machine Learning in Healthcare
- Improved Accuracy
Machine learning algorithms can analyze vast amounts of data far more efficiently and accurately than humans, helping reduce diagnostic errors. By identifying patterns and anomalies that may be missed by the human eye, machine learning models help provide more accurate diagnoses.- Example: In oncology, AI models can analyze tissue samples and Diagnosis with Machine Learning images to detect early signs of cancer, increasing the chances of successful treatment.
- Faster Diagnoses
Time is of the essence in healthcare, and delays in diagnosis can lead to worse patient outcomes. Machine learning models can process data rapidly, providing near-instantaneous results. In areas like radiology and pathology, where timely diagnoses are critical, Diagnosis with Machine Learning can reduce the time it takes to detect conditions, facilitating faster interventions. - Cost Efficiency
Early and accurate diagnoses lead to better treatment outcomes and reduced healthcare costs in the long run. By reducing misdiagnoses, unnecessary tests, and treatments, machine learning helps healthcare providers optimize their resources and improve operational efficiency.- Example: With AI-powered diagnostic tools, hospitals can automate repetitive tasks, such as image analysis, freeing up healthcare professionals to focus on more complex cases.
- Personalized Healthcare
Machine learning allows healthcare to be more personalized by analyzing data specific to individual patients. From genetic data to lifestyle choices, Diagnosis with Machine Learning models provide insights that help tailor treatment plans to the unique needs of each patient, improving the likelihood of a positive outcome.- Example: In oncology, ML-driven precision medicine can help identify the most effective chemotherapy drugs based on a patient’s genetic makeup, improving survival rates and reducing side effects.
- Continuous Improvement
Machine learning models improve over time as they are exposed to more data. As more patient data is collected and analyzed, these models become more refined, improving their diagnostic capabilities. This iterative learning process helps enhance the accuracy of Diagnosis with Machine Learning and ensures that healthcare providers stay at the cutting edge of medical advancements.
Challenges of Diagnosis with Machine Learning in Healthcare
While machine learning offers immense potential in healthcare, there are challenges to overcome:
- Data Privacy and Security: Healthcare data is highly sensitive, and ensuring that patient information remains secure is a top priority. Machine learning models must be designed to comply with regulations like HIPAA and GDPR, ensuring that patient privacy is protected.
- Data Quality and Availability: Machine learning algorithms require high-quality, well-structured data to produce accurate results. In some cases, healthcare systems may have incomplete or inconsistent data, which can hinder the performance of ML models.
- Integration with Existing Systems: Integrating machine learning models with existing healthcare IT systems, such as electronic health records (EHRs) and diagnostic equipment, can be complex. Ensuring seamless integration is critical for the widespread adoption of machine learning in healthcare.
- Regulatory and Ethical Concerns: The use of AI and Diagnosis with Machine Learning in healthcare raises ethical and regulatory questions. Ensuring transparency in ML decision-making processes, validating algorithms for clinical use, and ensuring human oversight are crucial to building trust in these technologies.
The Future of Machine Learning in Healthcare Diagnosis
As Diagnosis with Machine Learning technology continues to evolve, its role in healthcare diagnosis will expand. The future holds immense promise, with the potential for even more accurate, timely, and personalized diagnoses. We can expect further integration of machine learning with other cutting-edge technologies, such as genomics, wearable devices, and robotics, creating an ecosystem where healthcare is more proactive, preventative, and tailored to individual needs.
In the coming years, AI-driven tools will likely become commonplace in medical practices, empowering healthcare providers to offer better, faster, and more personalized care, ultimately leading to improved patient outcomes and a healthier society.
Final Thoughts: A New Era in Healthcare
Diagnosis with Machine Learning is revolutionizing healthcare by improving diagnostic accuracy, enhancing patient outcomes, and making healthcare more efficient and personalized. As the field continues to evolve, machine learning will play a central role in transforming healthcare, enabling faster, more accurate diagnoses, and ensuring that treatment plans are tailored to the individual needs of each patient. The integration of Big Data and machine learning into healthcare is not just improving diagnoses; it is reshaping the entire healthcare landscape, offering a brighter, healthier future for patients worldwide.