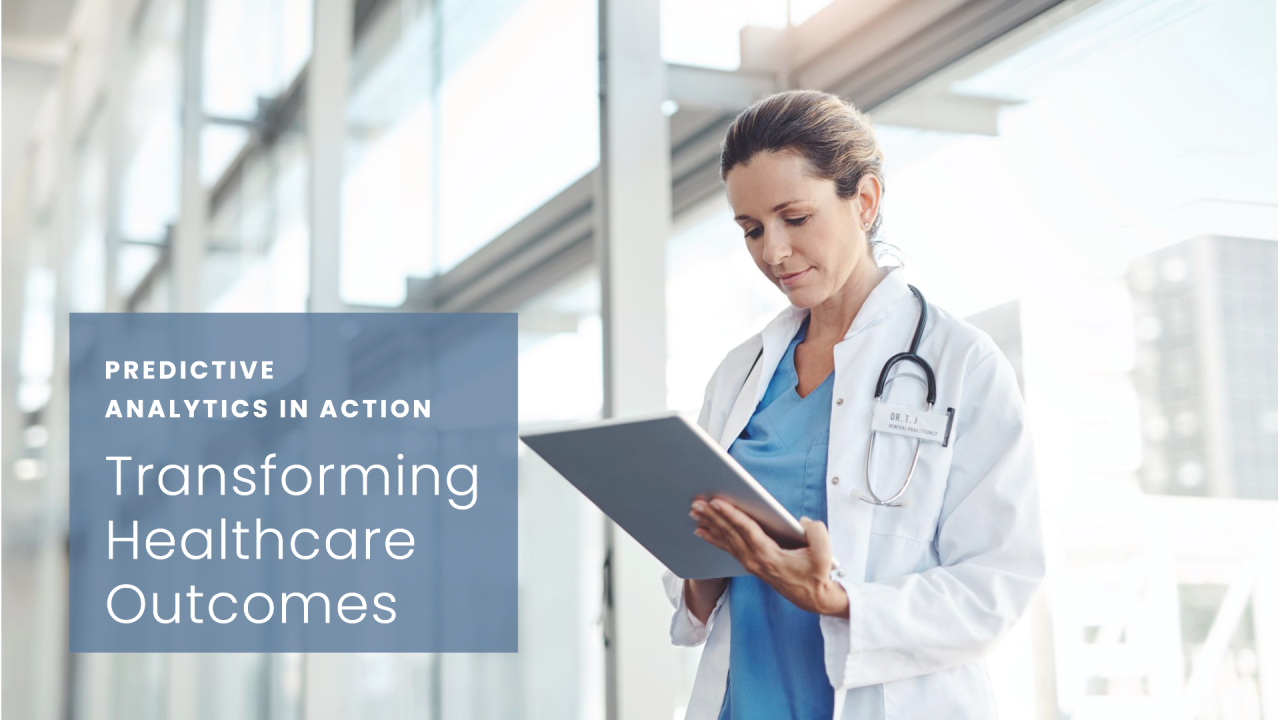
For Every Business, where data is being generated at an unprecedented rate, the healthcare industry is increasingly turning to predictive analytics to improve patient outcomes. Predictive analytics involves using historical data and statistical algorithms to forecast future events, enabling healthcare providers to anticipate patient needs and intervene before health issues escalate. This shift from reactive to proactive care is reshaping the way we think about healthcare, and it’s already making a significant difference in the lives of patients around the world.
Table of Contents
What is Predictive Analytics in Healthcare?
Predictive analytics in healthcare refers to the use of data mining, machine learning, and statistical algorithms to predict the likelihood of future events based on historical and real-time data. In healthcare, this typically involves analyzing a wide range of patient data — such as medical history, lifestyle habits, lab results, and genetic information — to forecast potential health risks, disease progression, or treatment outcomes. (Ref: Big Data Applications In Healthcare And How They’re Changing Lives)
By harnessing the power of predictive analytics, healthcare providers can move beyond merely responding to health problems and begin taking action to prevent them before they arise, ensuring more personalized and timely care for patients.
How Predictive Analytics is Revolutionizing Proactive Patient Care
- Early Identification of High-Risk Patients: Predictive models use data to identify patients at high risk for chronic diseases such as diabetes, heart disease, or stroke. By analyzing patterns in past medical records, healthcare providers can pinpoint individuals who are at greater risk based on factors like family history, lifestyle choices, or previous health conditions.
- Impact: Early identification allows for earlier intervention, such as preventive screenings, lifestyle modifications, or medications, all of which can dramatically reduce the risk of developing serious health conditions. This not only improves patient outcomes but also reduces healthcare costs by preventing hospitalizations and complications.
- Managing Chronic Conditions Effectively: Chronic diseases are among the leading causes of death and disability worldwide. Predictive analytics can play a crucial role in helping healthcare providers monitor patients with chronic conditions like asthma, diabetes, or hypertension. By continuously analyzing data from patient monitoring devices, wearables, and electronic health records (EHR), predictive models can identify early warning signs of deteriorating health.
- Impact: When healthcare providers receive alerts about changes in a patient’s condition — such as fluctuating blood sugar levels or irregular heartbeats — they can intervene promptly to adjust treatments or provide necessary support. This reduces the likelihood of severe complications and emergency room visits, allowing patients to live healthier, more fulfilling lives.
- Optimizing Treatment Plans: Predictive analytics doesn’t just help in identifying risk factors; it also assists in personalizing treatment plans. By analyzing vast amounts of clinical data, healthcare providers can identify which treatments are likely to be the most effective for individual patients, taking into account their unique medical history, genetics, and lifestyle.
- Impact: Tailoring treatment plans to each patient’s specific needs leads to better outcomes and fewer adverse side effects. It eliminates the trial-and-error approach often seen in traditional healthcare, making the entire treatment process more efficient and effective.
- Reducing Hospital Readmissions: Predictive analytics can also help reduce hospital readmissions — a key goal for healthcare providers, as frequent readmissions are costly and can negatively impact patient health. By analyzing data from previous hospital stays, predictive models can forecast which patients are most likely to be readmitted and what factors contribute to their risk.
- Impact: With this information, healthcare providers can take proactive steps, such as scheduling follow-up appointments, providing at-home care, or adjusting discharge instructions to ensure that patients adhere to their treatment plans. Reducing readmissions not only improves patient well-being but also lowers the burden on healthcare systems.
- Enhancing Preventative Care: One of the most powerful aspects of predictive analytics is its ability to drive preventative care. By analyzing trends and risk factors in patient populations, healthcare providers can identify individuals who may benefit from preventative interventions, such as vaccination, screenings, or lifestyle changes.
- Impact: Early prevention can significantly reduce the incidence of serious health conditions, from cancer to cardiovascular diseases. Predictive analytics ensures that patients receive the right preventive measures at the right time, contributing to longer, healthier lives and reduced healthcare spending.
Real-World Examples of Predictive Analytics in Action
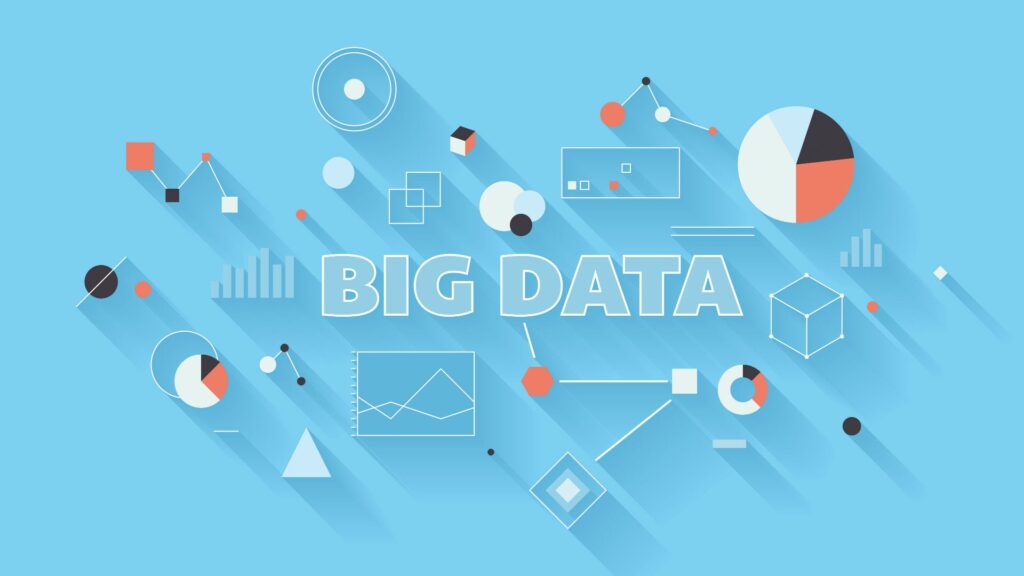
- Sepsis Prediction: In hospitals, predictive analytics is used to identify patients at risk of developing sepsis, a life-threatening condition that can arise from infections. By analyzing patient vital signs and lab results, predictive models can flag early signs of sepsis, allowing for rapid intervention and treatment, which can save lives.
- Readmission Risk Models: Hospitals and healthcare providers are increasingly using predictive models to assess the likelihood that a patient will be readmitted after discharge. These models take into account a variety of factors, including age, the severity of illness, and social determinants of health, to help healthcare teams develop more effective discharge plans and follow-up care.
- Chronic Disease Management: Health systems like the Mayo Clinic and Cleveland Clinic use predictive analytics to manage chronic diseases. For instance, diabetes patients who regularly upload data from their glucose monitors can be monitored in real-time. Predictive models alert healthcare providers to any alarming trends, allowing them to intervene before serious complications arise.
The Future of Predictive Analytics in Healthcare
The potential for predictive analytics in healthcare is vast. As technology continues to evolve and more data becomes available, predictive models will become even more accurate and widespread. The integration of artificial intelligence (AI) and machine learning into predictive analytics will enable healthcare providers to make real-time decisions, improving patient care even further.
In the coming years, predictive analytics could be integrated into the daily lives of patients through wearable devices, mobile apps, and telemedicine platforms, ensuring continuous monitoring and proactive care for a wide range of health issues. This approach promises to revolutionize healthcare, making it more personalized, cost-effective, and patient-centered. (Ref: Data Integration & Management in Big Data)
Final Thoughts : Empowering Proactive Patient Care
Predictive analytics is transforming healthcare by shifting the focus from reactive care to proactive, data-driven decision-making. By enabling early detection of health risks, personalized treatments, and better chronic disease management, predictive analytics is not only improving patient outcomes but also reducing healthcare costs. As healthcare systems continue to adopt these technologies, patients will experience more timely interventions, enhanced quality of life, and better overall health — making predictive analytics a true game-changer in the world of healthcare.