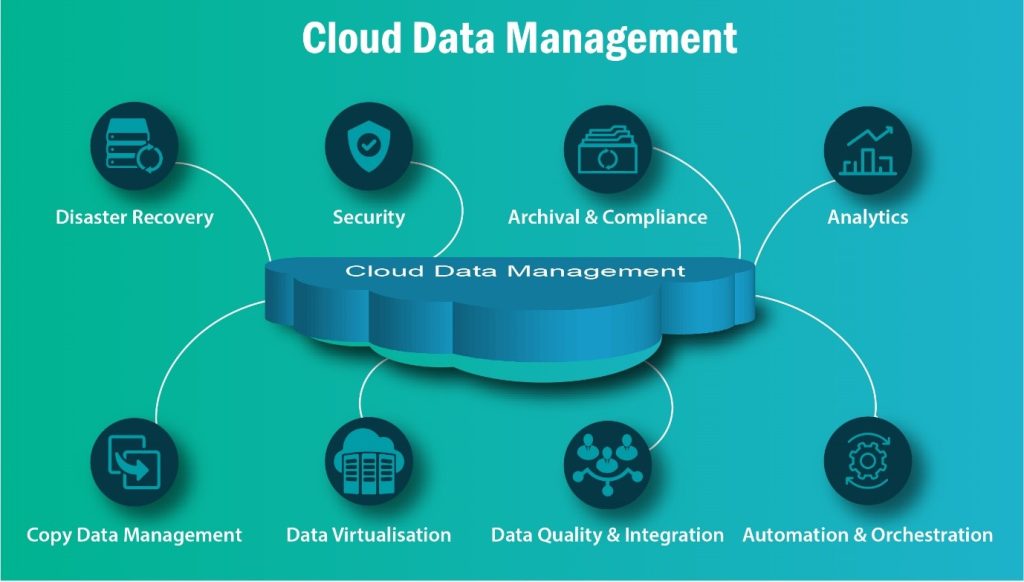
For Every Business, data is one of the most valuable assets for organizations across industries. From improving customer experiences to driving operational efficiencies and supporting business decisions, data is at the heart of everything businesses do. However, the true value of data can only be realized when it is accurate, consistent, and trustworthy. This is where data quality management (DQM) comes into play—ensuring that the data used across the organization is of the highest quality.
In cloud data warehousing, the ability to manage and maintain high data quality is critical. Poor data quality can lead to flawed insights, erroneous decisions, and costly operational inefficiencies. Fortunately, cloud data warehouses offer powerful tools and frameworks for data quality management, ensuring that organizations can rely on their data for accurate and actionable insights.
In this blog post, we’ll explore the importance of data quality management in cloud data warehousing, the challenges associated with it, and how organizations can implement best practices to ensure they get the most out of their data.
Outline
What is Data Quality Management (DQM)?
Data Quality Management is the process of ensuring that data is accurate, complete, consistent, timely, and relevant for its intended use. This includes managing and maintaining data quality throughout its lifecycle—from collection and storage to processing, analysis, and reporting.
Effective data quality management involves identifying and addressing data issues such as:
- Data Duplication: Identical data entries that can lead to confusion and inefficiencies.
- Data Inaccuracy: Incorrect or outdated data that compromises decision-making.
- Data Completeness: Missing or incomplete data that makes it difficult to gain full insights.
- Data Consistency: Conflicting data across different systems or sources.
- Data Timeliness: Ensuring data is up-to-date and available when needed.
In cloud data warehousing, DQM focuses on maintaining these standards at scale, enabling organizations to make data-driven decisions with confidence.
Why Data Quality Management Matters in Cloud Data Warehousing
1. Accurate Decision-Making
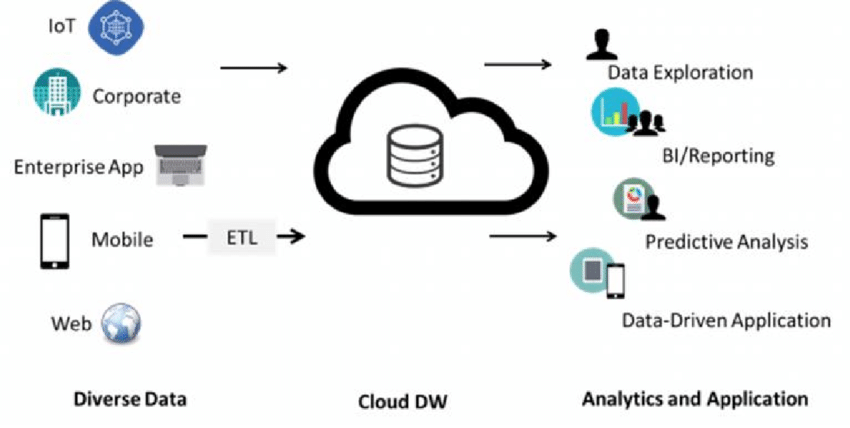
Data is the foundation of business decision-making. When data quality is poor, decisions made based on that data are likely to be flawed. A high-quality, clean dataset ensures that analytics and business intelligence (BI) tools provide accurate, actionable insights that help organizations make informed decisions. For example, sales forecasts, customer insights, and financial planning all depend on clean, reliable data to drive successful outcomes.
By implementing DQM processes in cloud data warehouses, businesses can trust the insights derived from their data, leading to more confident and accurate decision-making across all levels of the organization.
2. Enhanced Customer Experience
In today’s customer-centric world, delivering a seamless and personalized customer experience is paramount. Organizations rely on data to understand customer preferences, behaviors, and interactions. If that data is inaccurate or incomplete, it can lead to poor recommendations, missed opportunities, or even customer dissatisfaction.
Data quality management ensures that customer data stored in cloud data warehouses is clean, accurate, and up-to-date, enabling businesses to create targeted marketing campaigns, personalize content, and deliver the kind of customer service that fosters loyalty and satisfaction.
3. Operational Efficiency
Poor data quality can lead to inefficiencies across business operations. For example, inaccurate inventory data can lead to stockouts or overstocking, while inconsistent financial records can complicate reporting and auditing. By maintaining high data quality, organizations can streamline their processes, reduce errors, and eliminate the need for time-consuming manual corrections. (Ref: Unlock the Power of AI and ML Integration with Cloud Data Warehousing)
Cloud data warehouses provide a centralized platform for ensuring data quality at scale, reducing redundancy and the risk of errors that often come with managing data across disparate systems.
4. Compliance and Risk Management
In many industries, regulations require that companies maintain high data quality standards. For instance, data privacy laws like the GDPR (General Data Protection Regulation) mandate that businesses handle personal data accurately and securely. Poor data quality can result in compliance violations, legal penalties, or reputational damage.
By implementing robust data quality management practices in their cloud data warehouses, organizations can ensure they are compliant with regulatory requirements, mitigate risks, and avoid costly fines or legal complications.
5. Cost Reduction
Data quality issues often result in wasted resources—whether it’s fixing errors, handling discrepancies, or redoing analyses. By improving data quality upfront, businesses can reduce the time and cost associated with managing bad data, as well as avoid missed opportunities or misguided strategies.
Cloud data warehouses, with their scalability and flexibility, make it easier to implement DQM practices across large datasets, reducing the overall cost of poor data quality and enabling businesses to allocate resources more effectively.
Best Practices for Data Quality Management in Cloud Data Warehousing
1. Establish Data Quality Standards
The first step in data quality management is defining clear standards for data quality. These standards should align with business objectives and include metrics like accuracy, completeness, consistency, and timeliness. Setting these benchmarks helps ensure everyone in the organization understands what constitutes high-quality data.
Cloud data warehouses allow businesses to automate the monitoring of these standards and flag any data quality issues in real-time, ensuring ongoing data governance and quality assurance.
2. Data Profiling and Cleansing
Data profiling is the process of analyzing data to understand its structure, quality, and patterns. By profiling your data, you can identify inconsistencies, redundancies, and gaps that may affect data quality.
Cloud data warehouses often come with built-in data cleansing tools that help identify and correct errors, such as removing duplicates, standardizing formats, or filling in missing values. Implementing regular data cleansing procedures ensures that only high-quality data is ingested into the system.
3. Automate Data Quality Monitoring
Cloud data warehouses allow businesses to automate many aspects of data quality management, including monitoring, validation, and error detection. With tools like Amazon Redshift Spectrum, Google BigQuery, and Snowflake, businesses can set up automated workflows that continuously check data quality and alert stakeholders to any issues that require attention.
Automating these processes reduces the risk of human error and ensures that data quality is maintained across large datasets and multiple sources.
4. Data Integration and Consistency
Data often comes from multiple sources, and inconsistencies across these sources can lead to data quality issues. Cloud data warehouses are equipped with powerful data integration capabilities that can combine data from diverse systems, applications, and platforms into a single, unified view.
By ensuring that data from various sources is consistent and accurate before it enters the warehouse, businesses can avoid discrepancies and create a reliable foundation for analysis and reporting.
5. Data Governance and Ownership
Data governance refers to the policies and processes that ensure data is handled properly and responsibly across the organization. Assigning data ownership to specific departments or individuals ensures accountability for data quality, making it easier to track issues and maintain consistent standards.
In cloud data warehouses, robust governance tools allow businesses to monitor data access, ensure security, and enforce data quality standards throughout the lifecycle of the data.
Final Thoughts
Data quality management is a crucial component of modern cloud data warehousing. With high-quality data, organizations can make accurate, data-driven decisions, enhance customer experiences, and improve operational efficiencies. By implementing strong data quality practices such as profiling, cleansing, and automation, businesses can ensure that their cloud data warehouses deliver the insights they need to stay competitive.
Investing in data quality management doesn’t just safeguard your data—it unlocks its full potential, empowering your organization to thrive in a data-centric world. As cloud technologies continue to evolve, organizations that prioritize data quality will be best positioned to leverage the power of their data and achieve long-term success.