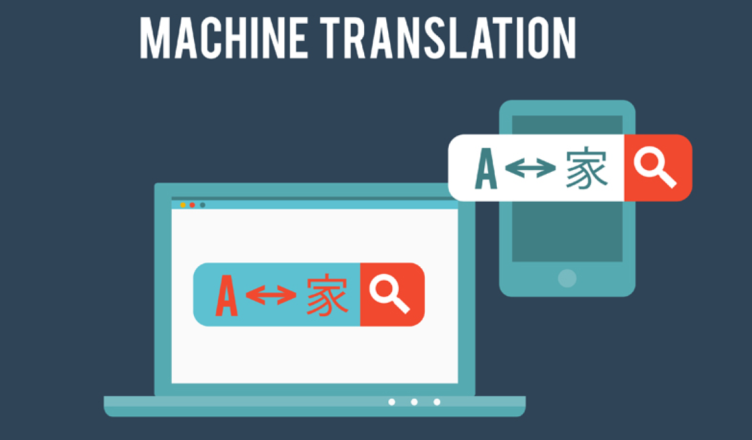
For Every Business globalized world, seamless communication across languages is more crucial than ever. Machine translation, powered by Natural Language Processing (NLP), is one of the groundbreaking technologies transforming how we connect, collaborate, and conduct business internationally. From translating a website for diverse audiences to facilitating real-time customer support in multiple languages, NLP-based machine translation is redefining cross-cultural interactions. Here’s a closer look at how NLP is driving advancements in machine translation, key techniques involved, and the future potential of this technology.
Outline
Understanding NLP in Machine Translation
Natural Language Processing is a branch of artificial intelligence that enables computers to understand, interpret, and generate human language. In MT, NLP algorithms convert text or speech from one language to another. The objective is to achieve translations that are not only accurate but also retain the original meaning and context, something that’s notoriously challenging for machines.
Key Applications of NLP in Machine Translation:
- Global Content Localization
Businesses today operate in international markets, making it essential to localize content for various audiences. Machine translation helps companies adapt their websites, products, and marketing materials to different languages and cultures, enabling them to reach a broader customer base. - Real-time Customer Support
NLP-powered is vital for companies offering multilingual customer service. Real-time translation helps bridge language gaps between support agents and customers, improving communication and ensuring a positive customer experience. - E-Learning and Educational Content Translation
Is transforming online education by making learning materials accessible in multiple languages. With accurate translations, students from different linguistic backgrounds can access valuable educational content, advancing their learning experience. - Social Media and Content Moderation
Is essential for monitoring global social media platforms, where users communicate in diverse languages. NLP translates user content, helping moderators understand sentiments, detect inappropriate content, and respond accordingly. - Cross-border Legal and Financial Communications
In sectors like legal and finance, where precise terminology is crucial, machine translation enables global communication while maintaining accuracy. By translating contracts, documents, and financial statements, NLP ensures clarity and compliance across regions.
Key Techniques in NLP for Machine Translation
Advancements in NLP in Machine Translation have introduced various models and techniques that improve the quality and reliability of machine translations. Here are some commonly used methods:
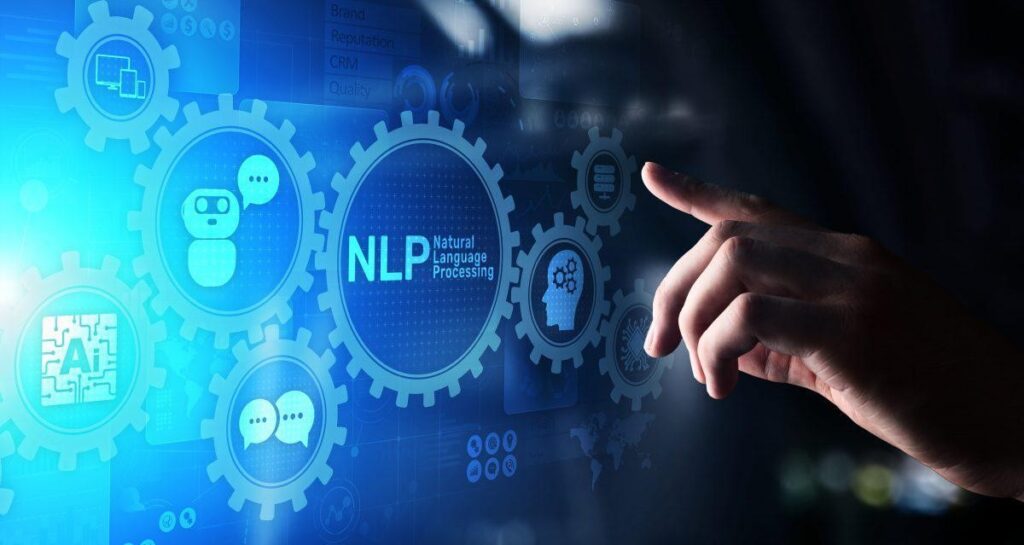
- Statistical Machine Translation (SMT)
SMT relies on statistical models to predict the probability of a sentence in the target language based on the source sentence. Although effective, SMT often struggles with contextual nuances and idiomatic expressions, leading to errors in complex translations. - Rule-based Machine Translation (RBMT)
In RBMT, linguistic rules are defined for both source and target languages, aiming to ensure grammar and syntax are preserved. However, this method can be limited by the vast number of rules required for different languages and scenarios. - Neural Machine Translation (NMT)
NMT, powered by deep learning, has become the most promising approach in machine translation. Unlike SMT, which translates word-by-word, NMT considers entire sentences or even paragraphs, producing more natural and fluent translations. NMT models such as Transformer-based architectures (e.g., Google’s BERT and OpenAI’s GPT) are particularly popular due to their ability to capture linguistic context and subtleties. - Hybrid Machine Translation
Some systems combine rule-based and statistical or neural approaches to leverage the strengths of each. Hybrid methods are beneficial in specialized fields like legal and medical translations, where accuracy is paramount, and a mix of rules and statistical predictions can enhance reliability.
The Role of Deep Learning and Transformers
The breakthrough came with the development of Transformer models, which introduced attention mechanisms to understand context better. The attention mechanism allows the model to weigh the importance of words within a sentence, resulting in more accurate translations. Popular models like GPT-3, T5, and BERT have transformed machine translation by providing nuanced, context-aware translations that are closer to human accuracy.
Benefits and Challenges of NLP in Machine Translation
Benefits:
- Faster and Scalable Translations: NLP enables large-scale translation projects to be completed in a fraction of the time it would take humans.
- Improved Accessibility: Machine translation helps break language barriers, promoting inclusivity and accessibility in global communication.
- Cost-effective: By automating translation tasks, businesses can significantly reduce costs compared to hiring a team of human translators.
Challenges:
- Context and Cultural Nuances: Language is often culturally specific, and struggles with idioms, humor, and regional dialects, which require an understanding of cultural context.
- Quality Assurance: While NLP has improved, human review is still essential for high-stakes translations where accuracy is critical, such as legal or medical content. (Ref: Natural Language Processing)
- Data Dependency: It models require massive datasets to improve accuracy. High-quality, multilingual datasets can be difficult to obtain for lesser-known languages, limiting translation quality.
The Future of NLP in Machine Translation
As machine learning and NLP continue to evolve, we can expect to become even more sophisticated. With developments in multilingual NLP models and continual improvements in Transformer architectures, the accuracy of translations will continue to approach human levels. Future advancements may also allow real-time translation that accounts for cultural nuances, dialects, and slang, making communication across languages truly seamless.
Moreover, NLP-powered has the potential to support endangered languages, preserving them for future generations by making them accessible to a broader audience. Companies and researchers are also exploring the use of machine translation to support humanitarian efforts, bridging communication gaps in crisis situations where rapid and accurate translations are critical.
Final Thoughts
NLP in Machine Translation in transforming global communication, offering a reliable solution to overcome language barriers. From enhancing business operations to expanding educational access, machine translation is empowering individuals and organizations worldwide. As technology advances, NLP will continue to bring us closer to a world where language differences no longer stand in the way of understanding and collaboration.
By investing in NLP in Machine Translation, companies can unlock new markets, improve customer satisfaction, and make meaningful contributions toward a connected, multilingual world.