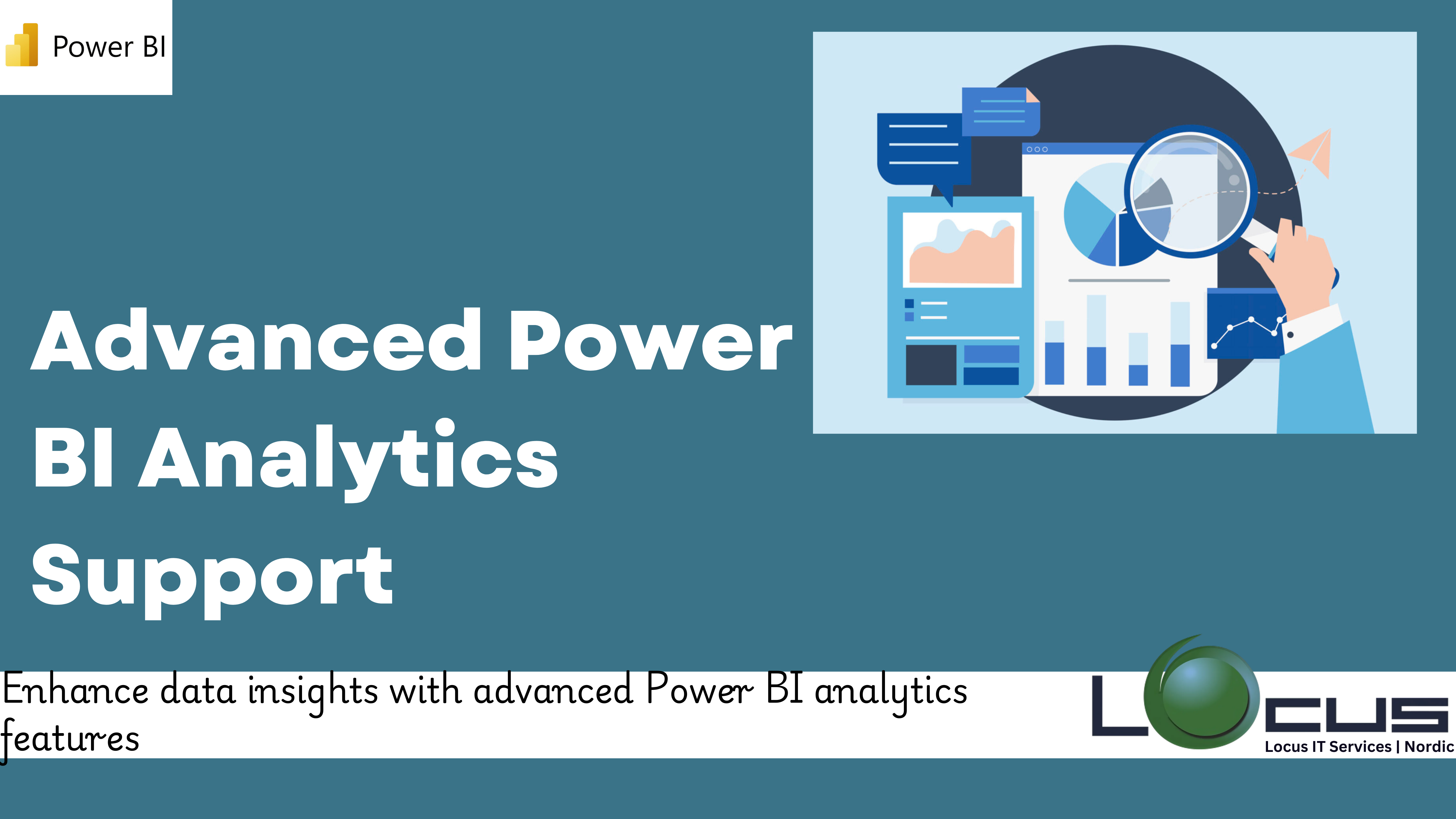
Microsoft’s primary business intelligence product, Power BI, has grown to be a crucial tool for companies trying to efficiently display and understand their data. Although Power BI’s primary functions include producing aesthetically pleasing dashboards and simple data reports, its sophisticated analytical features distinguish it as a revolutionary tool for extracting meaningful insights from data. Businesses can now use predictive and prescriptive analytics—which explain what will happen and how to achieve desired outcomes—instead of descriptive analytics, which explains what happened.
The importance, cutting-edge tools, advantages, and tactics for using Power BI analytics will all be covered in this in-depth conversation, which will assist organizations in realising the full potential of their data.
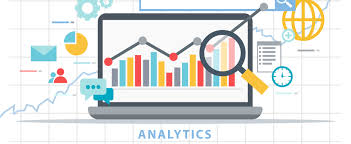
Why Use Power BI Analytics ?
Organisations must now derive useful insights from large datasets due to the changing data landscape. While Power BI analytics enable deeper capabilities like trend identification, future outcome forecasting, and the discovery of hidden patterns, traditional reporting frequently restricts users to historical analysis. This is made possible by Power BI’s strong interaction with machine learning, artificial intelligence, and sophisticated scripting languages like R and Python.
For businesses looking to make data-driven decisions that go beyond past trends, advanced Power BI analytics which is essential. (Ref: Power BI Data Visualization Troubleshooting)
Businesses can use it to:
Forecast Future Trends: To predict results, use forecasting and predictive models.
Find Hidden Patterns: To find insights, use correlations, anomaly detection, and clustering.
Make Better Decisions: Improve the accuracy of data interpretations to support strategic planning.
Automate Insights: Create actionable insights using AI-driven features to cut down on human labour.
Advantages of Power BI Analytics for Better Decision-Making
Businesses can rely on data-driven tactics to increase decision-making accuracy and agility by utilising forecasting and root-cause analysis tools.
Insights Automation: Power BI’s AI-powered solutions greatly save human labour and increase efficiency by automating the insights generation process.
Flexibility and Scalability: Because of Power BI’s smooth integration with Microsoft Azure, businesses can handle streaming data, do extensive Power BI analytics, and incorporate data lakes for big data scenarios.
Democratisation of Power BI analytics: Power BI makes it possible for non-technical people to examine and comprehend complicated information using features like natural language queries (Q&A) and user-friendly visualisation tools.
Important attributes of Power BI Analytics
1. R and Python integration: Users may run bespoke scripts for statistical analysis, machine learning, and data manipulation thanks to Power BI’s seamless integration with R and Python.
2. Models of AI and Machine Learning: Pre-trained models for text Power BI analytics, image recognition, and anomaly detection are among the AI elements that Power BI integrates. Custom models are also supported by Azure Machine Learning.
3. Forecasting and Analysis of Time Series: Power BI’s line and area charts come with built-in forecasting tools that let users predict future trends from past data.
4. Visual Decomposition Tree: By dissecting measures into contributing components, the Decomposition Tree enables users to conduct root cause analysis.
5. What-If Analysis: To examine the effects of various variables on results, Power BI allows what-if scenarios using parameters.
6. Segmentation and Clustering: You can segment your data or find trends by grouping data points according to similarities with Power BI’s clustering tool.
7. Identifying Anomalies: By assisting users in identifying anomalies in their data, Power BI’s anomaly detection feature facilitates the identification of problems or opportunities.
8. Natural Language Processing and Q&A: Non-technical consumers may now access Power BI analytics thanks to the Q&A function, which enables users to query their data in natural language.
9. Power BI has the ability to automatically produce narratives that clearly explain important insights.
The Best Ways to Use Power BI Analytics
Begin with clean data: Before using advanced Power BI analytics, make sure your data is correct, consistent, and organised.
Make Use of Outside Resources: For intricate computations and testing, use programs like DAX Studio, Python IDEs, or RStudio.
Employ filters and parameters: Add filters and parameters for dynamic analysis to make reports more interactive.
Integrate Features: For deeper insights, combine several advanced Power BI analytics methods (such as forecasting and clustering).
Record and Educate Users: Keep track of sophisticated features and teach people how to properly comprehend and interpret the results.
Problems with Advanced Analytics and Solutions
Complexity for Beginners: Start with Power BI’s built-in tools before advancing to Python, R, or Azure ML integration.
Performance Issues: Optimize your data model and use only necessary visuals to avoid slow report performance.
Interpretation of Results: Provide context and training to ensure stakeholders understand the insights generated.
Data Privacy: Use row-level security and adhere to compliance standards when sharing advanced reports.
Overcoming Challenges in Advanced Analytics
Complexity for Beginners: While Power BI simplifies analytics, beginners may find advanced features intimidating. Starting with built-in tools and gradually integrating R, Python, or Azure ML can ease the learning curve.
Performance Optimization: Reports with numerous visuals or large datasets can slow performance. To address this, users should optimize their data models and visuals.
Data Privacy: Row-Level Security (RLS) and compliance standards are vital for safeguarding sensitive data when sharing advanced analytics reports.
Interpretation of Results:
Providing clear context and explanations ensures stakeholders derive accurate insights from complex analytics.
Using Power BI to Future-Proof Advanced Analytics
Here are some techniques to prepare for the future of analytics with Power BI:
Ongoing Education: To take advantage of new advanced analytics features, stay up to date with Power BI’s weekly feature releases.
Interface with Data Lakes: For large-scale analytics and scalability, use Power BI’s interface with Azure Synapse or Data Lakes.
Cloud Integration: For real-time analytics with capabilities like incremental data loads and automatic page refresh, go towards hybrid or cloud-only data systems.
Future Directions for Power BI Advanced Analytics
Improved AI Integration: More predictive and prescriptive analytics will be possible with deeper integration with AI tools.
Real-Time Analytics: Real-time analytics will grow in strength and popularity with features like automatic page refresh.
Increased Self-Service Capabilities: Business users will be able to perform sophisticated analytics without the need for technical knowledge thanks to more approachable tools.
Augmented Analytics: AI will help users find important insights and make automatic recommendations for models or visualisations.
Final Thoughts
Power BI’s advanced analytics give users the means to glean more profound insights and arrive at well-informed conclusions. Organisations may go beyond descriptive analytics to predictive and prescriptive insights by utilising features like forecasting, clustering, machine learning models, and R/Python interaction.
Exploring advanced analytics will help you realise the full potential of your data, regardless of your level of experience with Power BI. This will help you remain competitive in a world that is driven by data.